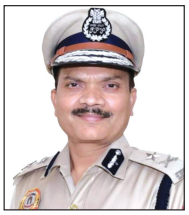
B Shanker Jaiswal, IPS, is the Joint CP Technology & Project Implementation in Delhi Police. His MS Research (MSR) thesis focused on predictive policing, culminating in the publication of two significant papers on crime prediction using AI methodologies. He is a proponent of leveraging cutting-edge technologies to enhance public safety.
He can be reached at sh********@***il.com.
Predictive policing is transforming law enforcement by harnessing artificial intelligence (AI) and machine learning (ML) to anticipate criminal activities. This approach allows for more efficient resource allocation and crime prevention. This article delves into the mechanics, applications, and impact of predictive policing on public safety, while also addressing the accompanying ethical and cybersecurity challenges. With real-world examples from global and Indian contexts, it highlights how predictive policing could revolutionise crime-fighting, despite hurdles like bias and data security concerns.
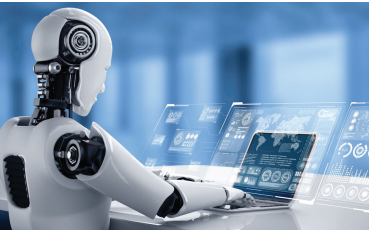
Understanding Predictive Policing
Predictive policing is rooted in applying data analytics to forecast crime hotspots or predict when and where crimes are likely to occur. This modern law enforcement tool analyses historical crime data to identify patterns, trends, and anomalies, which help police deploy resources more effectively. The focus is not on predicting specific criminal actions, but on determining the likelihood of crime in particular areas or by certain individuals.
Predictive policing operates similarly to weather forecasting: it provides probability-based estimates that guide decision-making. The key difference from traditional law enforcement is that predictive policing shifts from reactive to proactive. By anticipating crime, law enforcement agencies can deter criminal activities before they happen.
The predictive models utilize crime data, neighborhood characteristics, socio-economic factors, and real-time data like traffic patterns or social media activity. This dynamic approach makes predictive policing a forward-looking tool especially useful in urban areas with high crime rates and resource constraints.
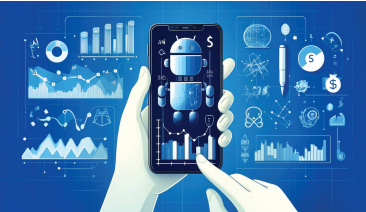
The Role of AI and Machine Learning
The evolution of predictive policing has been fuelled by advances in AI and ML. AI technologies, such as deep learning and neural networks, can process large datasets at incredible speeds, uncovering patterns often invisible to human analysts. In India and globally, this technological capability allows police forces to make sense of the overwhelming volume of data produced by modern cities.
Machine learning models are well-suited for predictive policing because they learn from data over time, which enhances their accuracy. For instance, when a model is trained on years of crime data, it can identify trends that repeat seasonally or correlate with specific events, such as festivals or political rallies. These AI models also incorporate real-time data feeds to improve predictions dynamically
In India, where the police face chronic manpower shortages, AI can enhance productivity by directing officers to areas where their presence is most needed. This technology is not just theoretical; cities like Delhi and Mumbai are already piloting AI-based predictive policing systems to manage crime more efficiently in densely populated urban areas.
Importance of Data and Technology
We are witnessing a pivotal moment in the intersection of data and technology. The availability of large datasets, enhanced computational power, and sophisticated algorithms have created unprecedented opportunities for police departments to make informed predictions about crime. This convergence enables law enforcement agencies to strategise and deploy personnel more efficiently, significantly improving their ability to prevent crime.
The potential of predictive policing is built on three pillars: data, computing power, and algorithms. Historical crime data provides a foundation for creating predictive models, while advanced AI techniques—such as deep learning and convolutional neural networks (CNNs)—enhance the precision of predictions. This synergy between data and technology makes it possible for police departments to make informed decisions about where and when crimes are likely to occur, improving public safety.
Data is the backbone of predictive policing. The reliability and accuracy of crime forecasts depend largely on the quality of data input. Traditionally, this data includes crime reports, arrests, and complaints, but modern predictive policing systems integrate additional layers like geographic data, CCTV footage, and even social media analysis.
In India, the police forces’ reliance on manual data collection has been a limiting factor. However, the advent of digital tools and initiatives like the Crime and Criminal Tracking Network & Systems (CCTNS) has streamlined data collection and sharing across various jurisdictions. This data centralisation is a significant step towards more robust and reliable predictive models.
Globally, advanced policing systems use big data analytics AI-powered algorithms to generate crime predictions. For instance, the Los Angeles Police Department (LAPD) employs PredPol, an AI system that analyses historical crime data and identifies high-risk areas. The software focuses on small geographical zones, guiding police patrols based on daily predictions.
Similarly, predictive policing models in countries like the UK have been employed to track potential terror threats and gang activities. AI integration with law enforcement practices ensures that data is analysed not just for trends but also for deep insights into criminal behaviour.
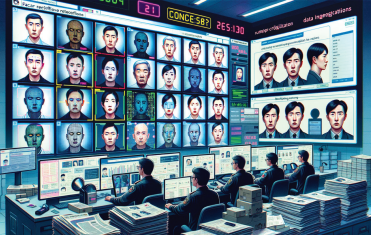
Applications of Predictive Policing
The applications of predictive analytics in policing extend beyond identifying potential crime hotspots. These tools are useful in numerous areas:
1. Crime Prevention: Police can focus their efforts on areas with the highest probability of criminal activity. By concentrating patrols and resources, agencies can prevent crimes before they occur.
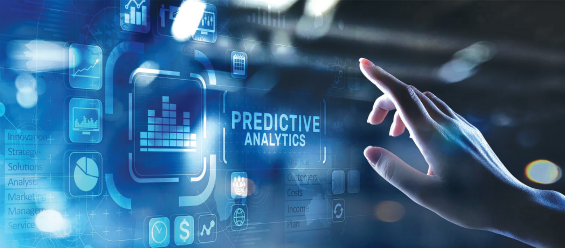
2. Operational Planning: Predictive models assist in planning large-scale operations like raids or controlling public events. For example, during political rallies or religious festivals in India, police can use predictive models to pre-emptively allocate resources to areas where unrest or criminal activity is expected.
3. Counter-Terrorism: Predictive policing has proven invaluable in combating terrorism using behavioural and geographic patterns to identify potential threats. In India, where terrorism remains a significant concern, the ability to predict extremist activities based on historical data can help law enforcement prevent attacks.
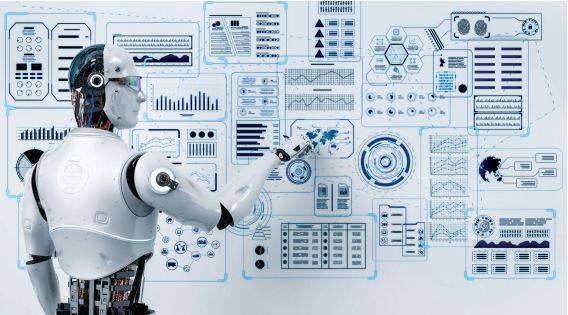
4. Traffic Management and Crime Detection: Some cities have integrated traffic and crime data to predict where accidents or crimes like robbery may coincide. In India’s metropolitan cities, integrating predictive models into Intelligent Traffic Management Systems (ITMS) is proving to be a vital step toward reducing both crime and traffic fatalities.
Predictive policing also holds promise for combating terrorism and gang-related offences. By analysing patterns in historical data and identifying at-risk individuals or groups, predictive models can guide interventions that prevent these crimes from escalating. This proactive approach has demonstrated its potential to reduce crime rates significantly across various urban centres.
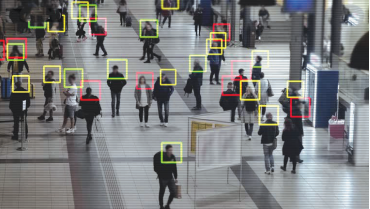
Mechanics of Predictive Models
The predictive policing models follow a well-structured process involving several stages. First, crime data from multiple sources is gathered, cleansed, and analysed. This data typically includes the location of crimes, times, types of offences, and socio-economic factors that influence crime rates.
Predictive policing relies heavily on algorithms that analyse historical crime data to identify trends and predict future incidents. These models are trained using crime data spanning several years—typically three to five years—to detect recurring patterns. For instance, in a large city like Delhi, predictions can be made for every day of the year, enhancing the precision and effectiveness of policing efforts.
The process begins with feeding the model historical data, such as crime location, time of occurrence, and socioeconomic conditions. Once trained, the model can forecast high-risk areas and suggest locations for increased patrols and law enforcement presence. These models are continually tested and refined, ensuring they remain accurate and relevant in dynamic urban environments. When law enforcement applies the insights generated by these models, they can prevent crimes before they happen and reduce crime rates significantly.
In practice, law enforcement agencies utilise block diagrams to train their models, test them, and ultimately make predictions. A critical outcome of this process is the identification of crime hotspots—specific areas where certain types of crime are most likely to occur. For example, in a city like Delhi, the model may generate predictions for 1,000 potential crime locations daily which are distributed across police precincts for targeted patrolling. By focusing on these predicted hotspots, police officers can utilise limited resources.
In Delhi, for instance, law enforcement agencies have historical data from thousands of reported incidents. Predictive models process this data using AI algorithms to identify crime hotspots and forecast patterns. Police officers receive daily forecasts with maps marking potential crime zones, helping them optimise their patrolling strategies.
The success of these models depends on constant refinement. As more data is input, the system ‘learns’ and adjusts its predictions accordingly. This iterative process ensures that the models stay relevant and accurate even in the face of changing crime dynamics, population shifts, and urban expansion.
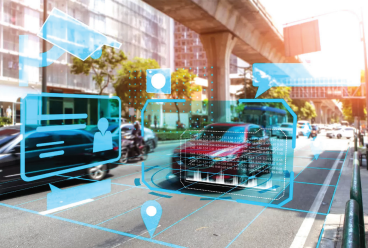
Global and Indian Context: Real-World Impact
Globally, predictive policing has shown positive outcomes. The previously mentioned trial in Los Angeles, where AI-driven patrol deployments reduced burglary rates by 17%, is a significant case study of its potential impact. Similar successes have been recorded in the UK and several European countries where predictive policing models are integrated into regular law enforcement practices.
In India, where police resources are stretched thin, predictive policing has great potential to increase efficiency. While still in its nascent stages, several Indian States are exploring pilot programmes to implement predictive analytics in routine policing. The Delhi Police’s initiative to utilise data analytics and AI for crime prevention highlights the growing trend of digital transformation aimed at enhancing public safety. Implementing these technologies, particularly in fast-growing cities like Bengaluru and Hyderabad, can effectively address challenges related to urbanisation and crime.
Addressing Cybersecurity Concerns
Despite its many advantages, predictive policing faces ethical and cybersecurity challenges that cannot be overlooked. One major concern is the vulnerability of predictive models to manipulation by malicious actors. Criminals could exploit these systems by feeding false data into the models or evading detection through sophisticated countermeasures.
Cybersecurity is paramount in maintaining the integrity of predictive policing systems. In India, the integration of AI in law enforcement makes these systems a target for cybercriminals. Police departments must implement robust encryption methods, secure data storage, and real-time monitoring to prevent breaches that could undermine public trust.
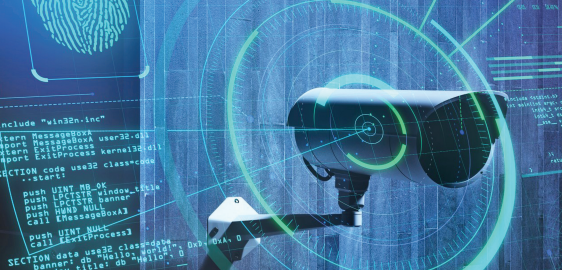
Ethical Considerations
While the benefits of predictive policing are clear, ethical concerns must be addressed. Using AI in law enforcement raises questions about privacy, bias, and accountability. For example, some predictive models have been criticised for reinforcing racial or socio-economic biases present in historical crime data. These biases can lead to over-policing in certain communities, undermining trust between law enforcement and the public.
Ethically, predictive policing raises concerns about bias. Historical crime data often reflects existing socio-economic disparities, leading to the over-policing of marginalised communities. For instance, some models in the U.S. have been criticised for racial bias, as they disproportionately target minority groups based on historical arrest records. In the Indian context, such bias could manifest in geographic or caste-based targeting, necessitating a careful review of algorithms to ensure fairness and transparency.
Robust cybersecurity measures are critical to safeguard predictive policing models. Ensuring data integrity, securing algorithms from tampering, and protecting individuals’ privacy are all essential for maintaining public trust in predictive policing technologies. Any breach of these systems could erode confidence and compromise their effectiveness, making cybersecurity a priority for all police departments implementing predictive models.
To ensure that predictive policing is implemented ethically, law enforcement agencies must prioritise transparency and fairness in their algorithms. Policymakers, data scientists, and law enforcement professionals must work together to develop frameworks that mitigate bias, protect individual privacy, and hold law enforcement accountable for how predictive models are used.
Interdisciplinary Nature of Predictive Policing
Predictive policing is inherently interdisciplinary, blending elements from criminology, AI, statistics, and sociology. By combining these fields, predictive models gain a holistic view of crime dynamics. Criminology, for instance, provides insights into why certain crimes occur in specific locations, while AI aids in identifying patterns invisible to human analysts.
Traditionally, criminology has focused on understanding criminal behaviour through biological, sociological, and psychological factors. However, recent studies have increasingly recognised the role of environmental factors—such as neighbourhood layout, population density, and economic conditions—in influencing crime rates.
In India, the traditional focus on crime prevention has relied heavily on sociology and psychology, but the integration of AI allows for a more data-driven approach. The interdisciplinary nature of this technology helps law enforcement understand crime from multiple angles, enhancing its ability to address complex challenges such as terrorism, organised crime, and cybercrime.
By integrating insights from criminology and data science, predictive models become more refined and accurate. This multidisciplinary approach enhances the ability of law enforcement agencies to predict criminal activity and take pre-emptive actions. Data mining, machine learning, and deep learning techniques play a crucial role in developing these models, ensuring that predictions are based on comprehensive and multi-dimensional analyses.
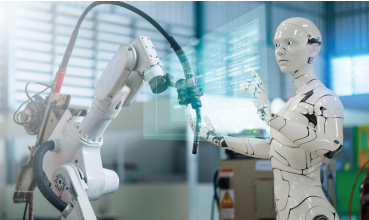
The Future of Predictive Policing
Looking forward, the role of AI in law enforcement is only expected to grow. Future predictive policing models will likely incorporate more real-time data sources, including social media monitoring, satellite imagery, and internet-of-things (IoT) devices like smart city sensors.
In India, as smart city projects proliferate, the integration of predictive policing within smart infrastructure will be critical. AI-driven surveillance, combined with IoT and traffic data, could help police respond more quickly to crimes and emergencies, making cities safer. This convergence of technology presents an opportunity for India to leapfrog into the next generation of law enforcement strategies.
However, with this future comes a need for greater oversight. Ensuring that predictive policing is used responsibly and ethically, particularly in diverse societies like India, requires legal frameworks that address privacy concerns and prevent misuse. Collaboration between technologists, law enforcement, and civil society will be key to navigating these challenges.
As technology continues to evolve, so too does the potential of predictive policing. AI and ML are poised to revolutionise law enforcement, shifting the focus from reacting to crimes after they occur to proactively preventing them. By combining predictive analytics with real-time data feeds, such as social media analysis and video surveillance, police agencies can respond more quickly and accurately to emerging threats.
Moreover, ongoing research into AI and ML will further improve the accuracy and reliability of predictive models. As new algorithms are developed, predictive policing will become more effective at preventing not only street crime but also complex criminal activities like terrorism and organized crime.
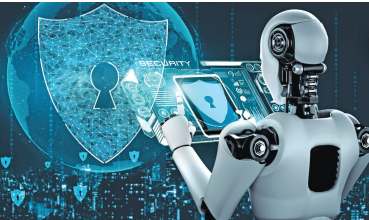
Conclusion
Predictive policing offers a powerful, data-driven approach to crime prevention, leveraging AI and ML to anticipate criminal behaviour. Its application in both global and Indian contexts highlights the potential for reducing crime rates and improving public safety. However, its success will depend on careful management of ethical concerns, cybersecurity risks, and the equitable application of algorithms.
In conclusion, predictive policing represents a significant advancement in crime prevention strategies, harnessing the power of AI and machine learning to forecast crime and allocate resources more effectively. By leveraging data-driven insights, law enforcement agencies can adopt a proactive approach to public safety, reducing crime rates and improving community well-being. As predictive policing continues to evolve, its integration into law enforcement practices promises a transformative impact. However, this evolution must be accompanied by a commitment to ethical standards and cybersecurity protections. As technology advances, the collaboration between data scientists, law enforcement professionals, and policymakers will be vital in addressing the challenges and opportunities that predictive policing presents. Predictive policing is not just a technological innovation; it is a societal shift that requires a balance between progress and responsibility.